- Published 19 May 2024
- Last Modified 19 May 2024
- 7 min
Machine Learning and Fault Diagnosis
Machine learning drives predictive maintenance by enabling analysis of sensor, maintenance and equipment performance data and preventing unscheduled maintenance and shutdown of machinery. Read our guide to find out more.
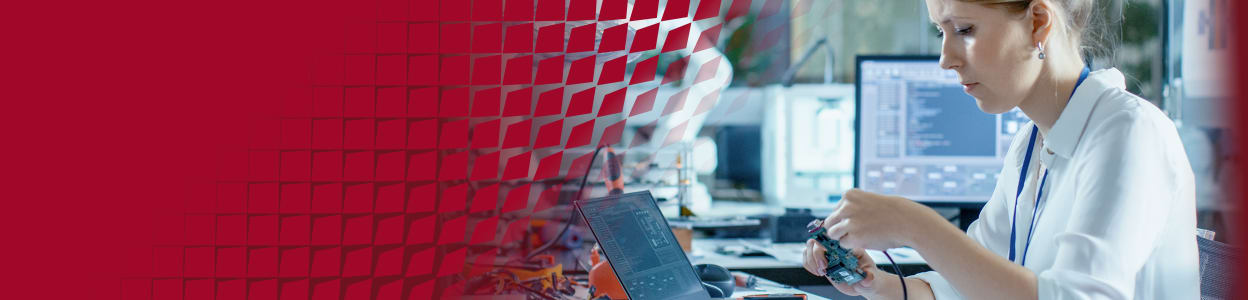
Reviewed by Allister Steel, Solution Engineer (April 2024)
Machine learning mimics human intelligence to solve problems and perform tasks. The technology is now proving its worth in analysing data for fault diagnosis and predicting failure as part of maintenance solutions. This guide provides an overview of fault diagnosis techniques, gives a quick introduction to machine learning, and looks at how machine learning is helping engineers predict failure.
Fault Diagnosis
Successful fault diagnosis is crucial to understanding where a piece of machinery is failing so that corrective action can be taken. In an industrial system, it’s necessary to isolate the fault, discover the type of fault, and pinpoint its location. Once a fault has been detected, engineers will also look to understand the root cause. They then carry out maintenance work to fix the fault and prevent it from happening again.
Machine learning integration with fault diagnosis is increasing the sophistication of fault diagnosis techniques and automating tasks – such as rapidly analysing very large volumes of data from sensors or industrial routers – that would be difficult for engineers to do. Machine learning integration is also enabling predictive maintenance by analysing data to predict faults before they occur, allowing engineers to intervene early.
Fault Diagnosis Techniques
In manufacturing, a number of failure analysis and fault-finding techniques and technologies are used for fault diagnosis. They include:
- Condition monitoring: Condition monitoring is typically used on rotating machinery and components like compressors, pumps, and motors. It involves monitoring a particular parameter – such as temperature changes – that may indicate a fault. Condition monitoring is a crucial part of predictive maintenance. Analysing minute variations in machine performance may indicate failure ahead of time and allow engineers to intervene
- Vibration monitoring: Vibration monitoring systems collect data from a network of sensors deployed on a machine. By measuring vibration, the system detects problems such as misalignment or loose parts in bearings, gears, shafts, and other components. Vibration monitoring is one of the most common forms of fault diagnosis techniques and helps enable predictive maintenance
- Oil analysis: Oil analysis involves taking samples of oil from machinery to assess its health and the health of the machine. For example, tiny particles of metal found in oil or other lubricants may indicate machinery wear. Engineers also measure the viscosity of the oil to check it is correct and to see if the oil needs replacement. This is crucial to the healthy functioning of rotating equipment
- Thermal imaging: Thermal imaging is used in fault diagnosis of electrical and electronic equipment. It works by detecting temperature changes. These changes could indicate that the equipment is overheating, is not cooling down at the desired rate, or has imperfections in its build
- Ultrasonic testing: Ultrasonic testing is one of a number of non-destructive testing (NDT) techniques for fault diagnosis. It works by sending ultrasonic waves through an object or material. The sound waves are transmitted to reveal flaws or characterise materials in sectors such as oil and gas, automotive, aerospace, construction, and medicine. A typical engineering application for it is ultrasonic thickness testing to assess the corrosion of pipework
Equipment for fault diagnosis includes cables and connectors. We also supply supporting electronics for condition monitoring like industrial ethernet switches, industrial wireless access points, industrial gateways, and industrial media converters.
What is Machine Learning?
Just what is machine learning? Machine learning is a part of artificial intelligence. Simply put, AI is the ability of a computer to mimic human intelligence. AI systems can thus solve problems and perform tasks similarly to people. Machine learning systems learn without following explicit instructions, e.g., programming. Engineers instead feed large volumes of data (such as data from sensors on machinery) and the machine learning model ‘trains’ itself to identify patterns or draw inferences from all that information.
Machine learning is particularly useful in situations with lots and lots of data – such as manufacturing sensor data. Machine learning can generate insights into that data in ways that may be difficult or impossible (or just too time-consuming) for people.
Diagnosing Faults with Machine Learning
Fault diagnosis using machine learning analyses very large volumes of data about machinery performance to identify problems. Deviations from the norm are identified by comparing data with historic trends. By identifying these deviations, machine learning can predict equipment failure before it occurs. This makes combining machine learning and maintenance a key enabler of predictive maintenance regimes.
Alerts
The predictive capabilities of machine learning can be used to analyse huge sets of data quickly. They also provide a warning to engineers to intervene if there is a problem. So, once a prediction of possible breakdown is identified, the system automatically alerts the engineer.
Predicting Failure
Predictive maintenance makes use of condition monitoring data from sensors to identify problems with performance that indicate a fault is developing. When combined with machine learning’s ability to analyse large amounts of data, the system can forecast breakdowns, helping prevent downtime and the need for reactive maintenance.
AI and Predictive Maintenance
With AI helping to increase the effectiveness of predictive maintenance alongside other aspects of Industry 4.0 such as the industrial IoT, Big Data, and automation trends, AI and maintenance are set to become more widespread as the smart factory of the future is realised. AI holds the promise of analysing not just the performance of individual machines but ultimately big networks of machinery to make maintenance decisions.
Benefits of Predictive Maintenance
Benefits of predictive maintenance include:
- Increased equipment uptime: Predictive maintenance helps avoid unplanned shutdown of machinery
- Improved productivity: Because engineers intervene to fix equipment before it fails, machinery is available for production for longer, boosting productivity
- Reduced costs: Running machinery to failure can be very expensive. Predictive maintenance addresses issues before they become serious and result in costly damage
- Better quality: Well-maintained machinery results in a high-quality production process
- Improved health and safety: Machinery breakdown can pose a hazard to workers. Identifying problems before breakdown can improve a facility’s health and safety
Benefits of Using Machine Learning for Maintenance
As a key part of AI, machine learning algorithms are the engines that facilitate predictive maintenance. They enable analysis of information that would otherwise be arduous for people, improve the performance of equipment, increase productivity, and prevent unscheduled reactive maintenance and shutdown of machinery.
The Future of Fault Diagnosis
Sophisticated predictive maintenance based on AI is more the exception than the norm right now. As this technology and its benefits become established, that is set to change. In the future machine learning and maintenance will become ever more entwined, which will reduce reliance on personnel, cut costs, and promise more accurate and efficient predictive techniques.
The future of machine learning itself will see engineers looking to develop algorithms that can work with smaller samples of data. They are also keen to explore the potential of machine learning to analyse a wider range of types of information – images as well as sensor data, for example – to improve maintenance.
Once predictive maintenance using AI is widely established, machine learning may be used to run complex workflows for an entire facility or even oversee machine operations completely independently of workers. For now, engineers will continue to exploit the growing potential of the technology to predict machinery failure well in advance.
Want to create a great predictive maintenance strategy? Read our guide here or visit RS Industria.