- Published 19 May 2024
- Last Modified 19 May 2024
- 8 min
Digital Twins and Maintenance
As virtual models of physical systems, digital twins are becoming more widely used in manufacturing. The twin is used to model everything from production processes and machinery to buildings, and assist with predictive maintenance, too. Our guide explains more.
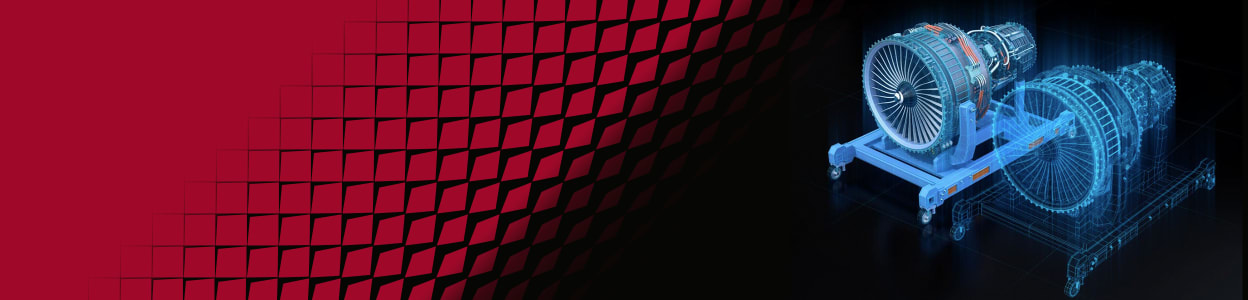
Reviewed by Allister Steel, Solution Engineer (April 2024)
Digital twin technology is a virtual representation of a physical object. That physical object could be a machine, product, vehicle, or process. Data is collected from the physical object and used to continuously update the digital twin. Digital twins are capable of running simulations and predicting future outcomes. For example, a digital twin can be used to predict the eventual end of an asset’s lifespan.
Digital twins also enable more efficient product development and help with predictive maintenance because they can predict machinery failure. In the future, they are expected to play a key role as the emerging field of prescriptive maintenance develops. Read on to learn more.
What is a Digital Twin?
So, what is digital twin technology? One digital twin definition is ‘a virtual model of a physical object.’ Types of digital twins may include sources of energy such as wind turbines or power plants, propulsion systems, or an entire car, aircraft, or factory. Manufacturers also use digital twins to visualise machine tools or create virtual manufacturing cells. Digital twins always feature a two-way flow of information between the physical object and the virtual model. That model is continuously updated with real-life data.
The digital twin can run simulations of the physical object’s behaviour. This information can then be used to make modifications to the physical system. Doing so helps optimise everything from R&D and design to manufacturing.
Benefits of Digital Twins
One of the key benefits of digital twin technology is that it’s possible to test changes to processes or designs without affecting the real-life system. Some digital twins can run lots of different simulations at once, enabling engineers to study numerous different outcomes. Engineers can then use information from the simulations to improve the design of a product or the manufacturing process. It might otherwise be very difficult to achieve this without disrupting operations or slowing down production.
Digital twins can also help speed up time to market by validating the design of a product before it exists, or identifying process failures before manufacturing starts. In this way, digital twins help identify risks, accelerate product development, and improve production processes.
During manufacturing, digital twins enable manufacturers to monitor and control product quality throughout the production process. By comparing virtual representations of products against design specifications and quality standards, manufacturers can detect defects, deviations, and anomalies early, ensuring consistent product quality and minimising the risk of defects, rework, and recalls.
Digital twins may be used to optimise the supply chain, providing visibility and connectivity at all stages from raw materials to finished product. By integrating data from suppliers, manufacturers, and customers, digital twins enable end-to-end optimisation of supply chain processes, including inventory management, production planning, and logistics, leading to reduced lead times, improved responsiveness, and enhanced collaboration.
Maintenance and Digital Twins
One of the other areas in which digital twins are having a big impact is maintenance. This is especially true when it comes to predictive maintenance.
Digital Twins and Predictive Maintenance
As well as helping with product development, digital twins are being used by manufacturers to model when physical systems will require repair. Notable digital twin technology examples of this include aero engine manufacturer Rolls-Royce using a digital twin to predict maintenance requirements for individual in-service jet engines.
A digital twin – much like a condition-monitoring system – relies on collecting large volumes of data from a machine. As data is captured, the model is continuously updated. Using the twin, engineers can visualise what is happening inside a physical system and plan maintenance accordingly. Capturing all that data can also help predict maintenance requirements or even the remaining life of the asset.
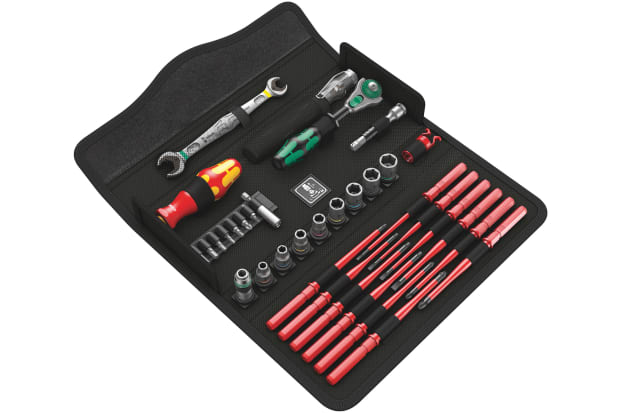
Digitalisation of Maintenance
Predictive maintenance is part of the increasing digitalisation of maintenance as a discipline. Predictive maintenance typically relies on employing networks of sensors to capture information about a machine and using AI or machine learning to assess any anomalies (in machinery vibration, for example) that indicate failure of a component, or complete breakdown of the machine, is imminent. That allows engineers to intervene before the problem gets worse.
Predictive maintenance results in less downtime and unplanned maintenance for the manufacturer, reducing costs and minimising lost production. Digital twins in maintenance are expected to make predictive maintenance more widespread. That’s because digital twins tend to rely on accurate data that can be used in a simulation to show where and when machinery failure might take place.
Of course, digital twins are just one technology in the digitalisation of maintenance. Other important aspects of this revolution include the use of computerised maintenance management systems (CMMS) to plan and schedule maintenance activities, and the use of the Industrial Internet of Things to collect data about machinery that feeds into maintenance regimes.
Digital Twins and Industry 4.0
The digital twin is a technology that is regarded as a key part of the fourth industrial revolution (Industry 4.0). Other key Industry 4.0 technologies include AI, machine learning, the IIoT, and Big Data. The fourth industrial revolution is marked by the convergence of physical and digital systems in a far-reaching digital transformation. With its virtual replication of machinery, entire products, or manufacturing processes, the digital twin is just one way in which this convergence is gathering momentum.
How Will Digital Twins Change Manufacturing?
Digital twins in manufacturing are expected to become a key enabler of accelerated product development by manufacturers. That’s because they allow engineers to make design changes and understand their impact without having to make costly and time-consuming alterations in the real world. When it comes to proving the design for a new product, the twin can also perform this job without the need to create a physical version.
In fact, interacting with and changing the digital twin is much easier than changing a product in real life. In this way, the twin provides a risk-free product development environment. Using digital twin technology in the initial stages of product development has the potential to enable engineers to work faster and cut costs. For manufacturing of complex, high-value, bespoke machinery, where it is difficult to produce a prototype for the customer, the twin also has the ability to iron out any problems using simulation rather than having to address issues once the machine is in service. Once a product has been launched, collecting real-world data and integrating it into the digital twin model can help make improvements.
As artificial intelligence and the IIoT continue to develop and become more widespread, the digital twin will also become more sophisticated. Digital twins may be used to simulate bigger systems such as entire cities or transport networks. As AI develops and automation trends progress, manufacturers will find themselves using digital twins that learn from experience to optimise manufacturing and offer new insights to improve product development.
Digital twin technology in construction is on the rise, too. Digital twins for the construction sector are based on real-life building or structure data collected using sensors or 3D scanners. The twin contains every aspect of the structure and its systems in a virtual environment. Digital twins for construction provide a means of improving building information modelling, making it more detailed and comprehensive. Similar to their manufacturing counterparts, construction teams can then experiment digitally and assess outcomes without making changes that impact the actual building or construction site.
One of the principal challenges of digital twins is maintaining the representation of the physical object correctly. The digital twin should reflect changes to the physical asset over time. That means engineers must make each digital twin include its asset’s operating condition changes and wear and tear. If the twin is not maintained effectively, then its usefulness goes down.
The Future of Maintenance
Future maintenance will see digitalisation continue and increasing levels of automation. For example, drones and robots will be used to conduct maintenance inspections in hazardous areas and perform tasks that are difficult or arduous for people to do. Predictive maintenance, whether via condition monitoring or the use of digital twins, is expected to become increasingly widespread. With advances in AI, maintenance systems could eventually learn from experience to self-diagnose failures in components and schedule work by automated technicians without human intervention.
In the long term, predictive maintenance is expected to evolve into prescriptive maintenance, where AI and machine learning make more and more sophisticated decisions. Prescriptive maintenance goes further than predictive systems because automation will not only predict failure, it will also identify the root cause while making recommendations for the best course of action for the engineer.
The smart factory of the future will have a much smarter maintenance regime to match.
Want to find out more? Develop your predictive maintenance strategy with the help of RS.